Google made a splash by announcing Med-PaLM2, a large language model fine-tuned for medical applications. The model achieved an impressive 85% accuracy on US Medical Licensing Examination (USMLE).
The results were quite remarkable. However, it was even more impressive that GPT-4, a general-purpose large language model, achieved a higher score of 86.65% without any fine-tuning.
Model fine-tuning is a highly complex process that requires expensive computing resources and extensive AI and domain knowledge. The fact that a general-purpose AI model could achieve performance comparable to that of a fine-tuned task-specific model was nothing short of revolutionary.
We are witnessing the greatest democratization of AI in the history of humankind.
Traditionally, most companies haven’t had the data, infrastructure, or expertise to train, deploy, and use AI models.
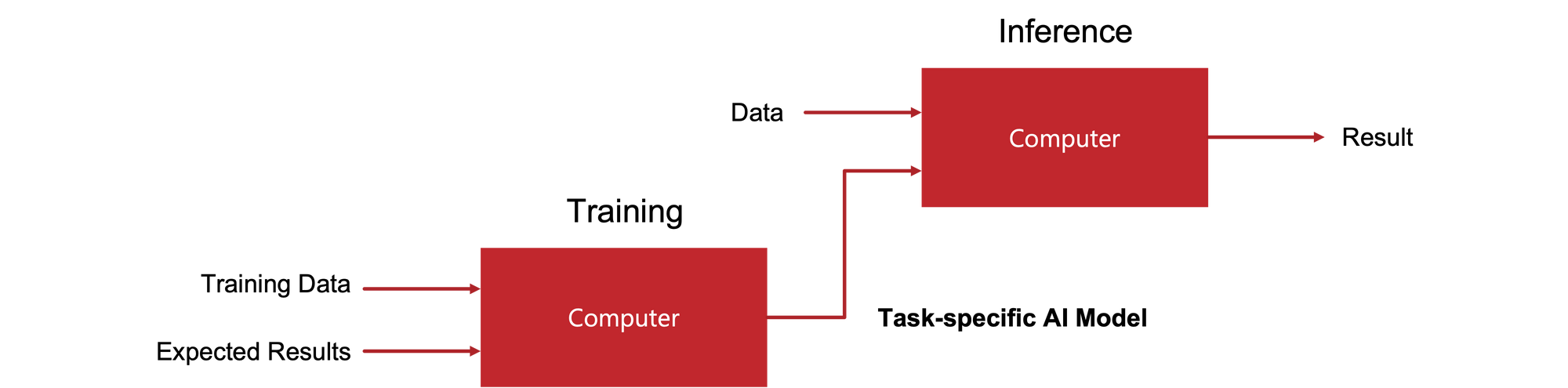
Data had to be extracted from disparate repositories, deduplicated, imputed, debiased, transformed, and annotated. AI models had to be trained, validated, deployed, and monitored.
An entire industry sprung up to facilitate this process. Yet, due to the effort required, only 10% of companies saw a significant return on their investments in AI.
Large foundation models dramatically change the ROI calculation.
A large foundation model is a platform that can be provided and consumed as a service with little to no customization.
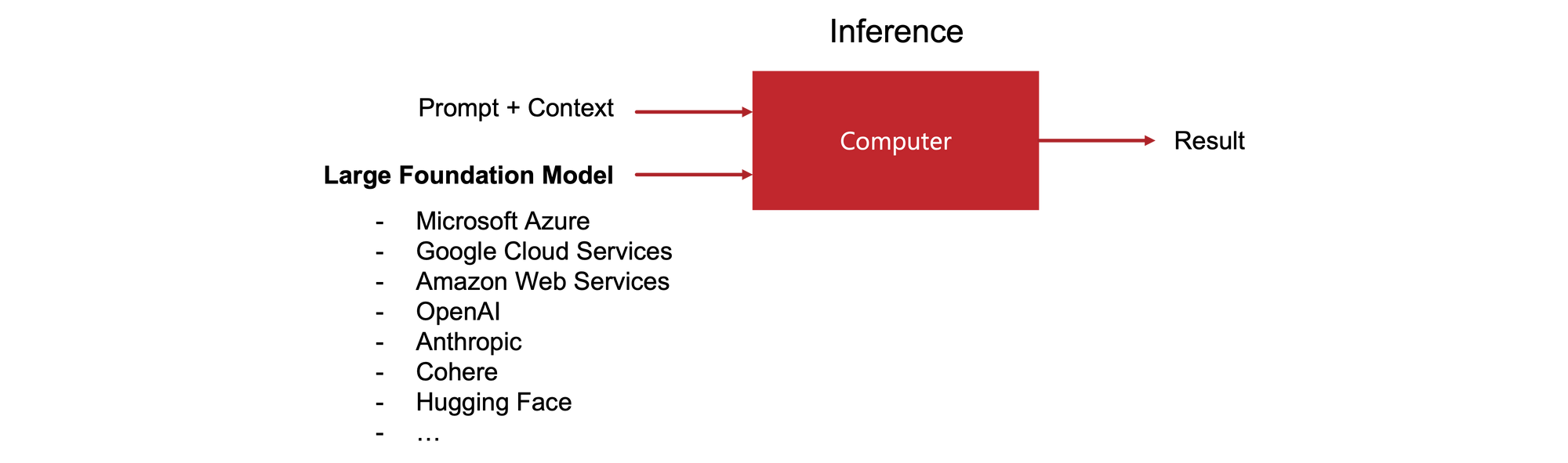
Suddenly, many business applications can be AI-enabled without the overhead of data preparation and model training.
As always, there is a catch.
It takes a lot of effort to train “traditional” task-specific models, but once trained, they provide predictable results. The same set of inputs produces the same set of outputs.
In contrast, large foundation models are inherently risky and unpredictable. They can hallucinate.
Therefore, we still need a layer that can make them suitable for deterministic, compliant, and task-specific business workflows. This function is performed by semantic brokers, a new category of enterprise software.
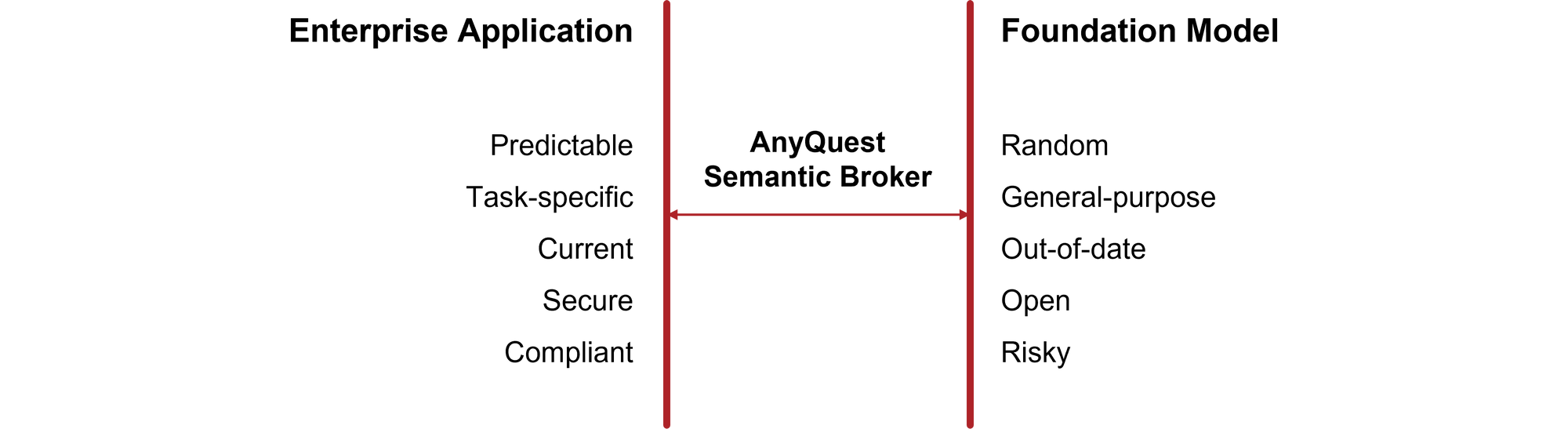